On-Demand Webinar
Low-code data engineering on Databricks for dummies
As organizations worldwide realize the transformative power of the Databricks Lakehouse to fuel their analytics and AI use cases, the need for efficient and reliable data pipelines has never been more crucial.
In this engaging webinar based on the content in the just-released Dummies book, we invite you to see how a low-code approach to data engineering can successfully democratize this function across your entire organization.
Watch as we demonstrate how Prophecy's low-code platform seamlessly integrates with the Lakehouse and empowers organizations to quickly deploy data-driven use cases with unparalleled ease and efficiency.
- A data engineering practitioner's perspective on how low-code transformed their business
- An architectural overview of how Prophecy’s low code platform and the Lakehouse enable data-driven, real-life use cases more easily
- A demo that illustrates how easy it is to build data pipelines for analytics and AI
Ashleigh
We have Mitesh Shah, who is Prophecy's VP of Market Strategy, helping simplify data transformation for all data teams here at Prophecy. Mitesh has 25-plus years of experience spanning roles, including product management, DBA, data scientist, and information security lead. Before Prophecy, Mitesh held positions as VP of product marketing strategy at Elation and MapR. He also holds an MBA from the Wharton School at the University of Pennsylvania and a degree in computer science from Cornell University. Welcome, Mitesh. Next up from the Prophecy team, we have Nathan Tong, who is a Sales Engineer who is committed to democratizing enterprise data to all data practitioners. Prior to joining Prophecy, Nathan was at Databricks for four years on the customer success engineering team, working with enterprise HLS retail and eLearning customers. Welcome, Nathan. And last but certainly not least, we're super excited to have Roberto Salcido, who is a senior solutions architect at Databricks. He loves helping customers build simple and elegant pipelines that enable downstream data analysts and data scientists to unlock the value of their data. Prior to Databricks, he was at Mode where he got a front row seat to the mainstream adoption of the modern data stack.
Ashleigh
In his free time, you can find him hiking Angel Island or watching a sports game with friends. With that, everyone, please join me in welcoming our speakers in the chat. Again, we're super excited to have you all here, so please introduce yourselves in the chat. Let us know where you're joining us from. Let's have a great session today. With that, I'd like to go ahead and hand it over to Mitesh to get us started. Mitesh, over to Outstanding.
Mitesh
Okay, thank you, Ashleigh. I appreciate it. Thank you, Nathan and Roberto, for joining. Thank you all for joining the webinar. I think it'll be a fun one. I wanted to start things off just by explaining a little bit about what we're going to talk about and how we're going to talk about it. This is intended to be, let's call it an approachable or non-intimidating guide to a couple of different concepts, including the Lakehouse as well as low-code data engineering. Many of you have probably seen the Low-code Data Engineering on Databricks for Dummies book, which we just showed a few slides ago. Perhaps you've downloaded it, flipped through the chapters. This is really meant to reinforce some of the content in that book, really in ways that we couldn't do on the written page. You'll see the demo here will be a big focus of the webinar today. Those are some of the goals. In terms of assumptions, we really aren't making many assumptions about where you're coming in from and where you are on your journey. I think the biggest assumption here is that you might be early or earlier in your low-code data engineering journey.
Mitesh
Perhaps you're just exploring solutions, perhaps you're just interested in the topic. Similarly, with the Lakehouse, maybe you're early in that journey as well. This is not meant to be a super duper advanced course on these concepts, but as you'll see from the demo, we will get into some great detail in just a bit. Last thing, and this is my favorite assumption, maybe you're new to some of these concepts, but we are certainly not going to assume that you are a dummy. The Dummies brand, it is a great brand for the book, but that's not the intention here. This is simply meant to convey that perhaps you're just new to some of these concepts. It's again meant to be an approachable guide to these concepts and one that is non-intimidating. In terms of agenda and flow for the webinar, we'll start with identifying the need for the Lake House and low-code data engineering. Roberto and I will cover that. Nathan will come in with the demo of Prophecy, so really stay tuned for that. It's going to be the hero of the next hour or so. It is what, summer or concluding the summer of 2023, we'd be remiss not to talk about the role of AI.
Mitesh
Ai does, in fact, play a very important role in both of our products. We'll talk a bit more about that later in the webinar. Then we'll conclude with what I'm calling tails from the field. We've got Roberto, we've got Nathan on the call, two folks that are talking to customers and prospects every single day about their pain, what they're feeling about the product. This will be a great opportunity to do a bit of Q and A with both Roberto and Nathan. With that, I'm going to now turn it over to Roberto to talk about the need for the Lakehouse. Roberto, take it away.
Roberto
Thanks, Mitesh, and thanks, everyone, for logging on here. I can see from the chat, it's a very global audience. So yeah, as Mitesh said, I'm going to talk through 100, 200 high-level value prop of the Lakehouse. Essentially, as this chart seems to indicate, most organizations are very advanced in terms of BI use cases and being able to analyze previous KPIs and represent that on a dashboard. But more and more in this day and age, customers are being asked to look into the future and do predictive modeling and try to ascertain what their business will look like three, four, or five years down the line, rather than what it looked like three, four, or five years previously. We call this the data maturity curve with the traditional use cases to the left and these more AI-driven predictive use cases on the right. Next slide, please. Great. But to actually actualize this vision, I think there might be some more content on this slide. Perfect. Thank you. The challenge with doing this is traditionally you would have two pieces of architecture for your data platform. You'd have your data warehouse for more your BI dashboard and use cases, SQL use cases, and then you'd maintain a separate data lake for your AI use cases, for your streaming use cases, for your unstructured data use cases.
Roberto
And this would lead to a lot of issues that would inevitably arise. Next slide, please. Great. Yeah, and some of the issues with having and maintaining these two platforms are highlighted below. So basically, duplicative data silos, right? You're carrying two copies of the data. You have incompatible governance frameworks with your traditional data warehouse being more so table ACLs and your traditional data lake being more file and BLOB directory rewrite file based access, and then just incomplete support for use cases. With the warehouse designed more for BI use cases, SQL based use cases, and the Data Lake more so meant for data science, streaming, unstructured data use cases. So you're maintaining multiple copies of the data. It's a complex environment, managing and maintaining these two disparate platforms, and just the cost and the performance is not what you want it to be. Next slide, please. That was obviously the really big motivation behind the Lakehouse paradigm and the Lakehouse platform. So unifying it and having one simple, performant, collaborative platform for your data engineering, streaming, ML and BI use cases, so complete support for all your range of use cases, a unified governance framework, and being able to handle data applications and just support the full range of use cases for your business, not only looking into the past and analyzing previous KPIs, but looking into the future and inferring what trends will look like multiple years down the road.
Roberto
And for us, the fundamental pillars of the Lakehouse are Unity Catalog, which is our unified governance framework and Delta Lake, which is the open source technology that really allows for this ability to have your full support for your data lake use cases on top of the warehousing use cases that were previously added maintain a separate platform for the performance, reliability and governance capabilities. Next slide, please. Okay, I think this is on to you, Mitesh.
Mitesh
Outstanding. Okay, Roberto, thanks for the primer here, the introduction around the need for the Lakehouse. I'm going to now pivot a bit and talk about the need for low-code data engineering really focused on transformations. The core principle here is that raw data is rarely suitable for immediate consumption. Many of you in the audience, I'm sure, have raw data sitting in operational data stores, in your CRM systems, in your ERP systems, coming in from sensors, files, you name it, you've got a lot of data. That raw data, however, is rarely suitable for immediate consumption to train your machine learning models or for your historical reporting and BI purposes. You need to take that raw data and you need to transform it. You need to enrich it. You need to create AI and analytics-ready, what we call data products out of it before you actually do the data science and BI reporting work with that data. That is effectively just a very high-level two-step process. The first step being bring that raw data over into the Lakehouse. There's a lot of tools out there in the market that can help with just that, focused on the extraction and load part of ELT.
Mitesh
Then the second step is really to curate that data. By curate, I mean transform that data and turn that data into AI and analytics-ready data products. There is a design pattern or an architectural pattern here called bronze, silver, and gold, otherwise known as multi hop pattern that Nathan will go into in a bit more detail in just a bit with the demo. But the idea here is that raw data sits in the bronze layer. You need to take that data, filter it, perhaps clean it a bit, going into the silver layer, and ultimately it moves on to the gold layer. Once you've aggregated that data and turned it into those data-ready data products that are ready for machine learning models and reporting purposes. That's the idea here. The core, again, here is that data transformations going from bronze, silver to gold is the key to building those AI and analytics-ready data products. Simple enough idea. Many of you are probably very familiar with that idea already. There are, broadly speaking, two options now for transforming that data in the historical world. One option is simply using code and/or scripts to do those transformations. By code and scripts, I mean potentially PySpark, potentially Scala, potentially SQL.
Mitesh
The other option is to leverage legacy low-code solutions to do those transformations. Again, one option, code, another option, low-code solutions. By low-code, I mean visual drag and drop interface. But there are challenges with each of those approaches. Let's take code and scripts first. The challenge number one is I talked about SQL just a moment ago. Sql is great, maybe a little bit more user-friendly. Maybe a lot more people know SQL than they do PySpark and/or Scala, but it is limited, and specifically it is limited to really working on relational data. It doesn't do so well for unstructured data. Second big issue here is that you have now a dependency on skilled coders, skilled data engineers. These data engineers, these coders are potentially in very limited supply in your organizations. This is bad for both the line of business folks who want data products and data-ready products to be able to do their BI reporting and create their machine learning models, et cetera. But they're now waiting for very limited resources in the organization to actually code those transformations and return those data products. Bad for them, but also bad for data engineers and the coders because now they're focused on minimizing their backlog and going through the backlog of requests.
Mitesh
They're not focused on higher value tasks, which we'll talk more about later, but those higher value tasks being things like creating actually the standards that others in the organization can actually use. Bad for both parties and really bad for the organization. Third big issue here with code and scripts is that it's missing a lot of capabilities. You have none of the surrounding frameworks around being able to orchestrate jobs around that code, visual lineage, deployment, search capabilities.
Roberto
It's.
Mitesh
Just code. You're missing a lot of the capabilities there. So problems with the coding approach and problems here now, let's talk about with the legacy low-code solutions. So in legacy low-code solutions, there is a few problems. Typically, they have great visual drag and drop environments, but underneath that, they turn those drag and drop environments and drag and drop environments into code that is proprietary to the tool itself. And that, in effect, locks you into that particular vendor. So that's problem number one. Problem number two, in some cases with these legacy low-code solutions, it's non-native performance. By non-native, I mean non-native to the cloud. In some cases, in fact, you have to bring that data onto your desktop or onto your laptop and then actually transform the data from there. In effect, you've got a world of small data, but not big data and certainly not cloud. The third big problem here is no support for DataOps. There is no ability to create tests, no ability for CICD, no ability to commit code to get, et cetera. All because, again, all this code is proprietary in nature and again, locks you in to that particular tools and environment.
Mitesh
Those are some of the challenges. Now, Prophecy is the low code platform that really addresses all of those challenges. We cater to both the data platform teams, the technical folks, the skilled folks that are skilled in coding, as well as the business data teams, allowing everybody and meeting everybody where they are in terms of their skills, providing both a low code environment for dragging and dropping, a visual environment for dragging and dropping what we call gems to create those data pipelines, which you'll see in just a moment, but as well turning that visual environment into open code, PySpark, Scala, and SQL, so that you can collaborate with potentially the more technical folks that can fine-tune those transformations if needed, and you've got the best of both worlds. This will be a big focus for Nathan in just a minute. I'm going to conclude here with a slide. I'm honestly showing this because I just love this spinning orb and it is mesmerizing, but no. I'm showing here my differentiators for prophecy, one being that it is complete. We're going to focus on transformations, but you can do orchestration, deployment, management of your jobs as well within prophecy.
Mitesh
You're going to see in just a moment what low-code means. It's both low-code and code, and so you'll see that in the demo. It is an open environment in that what I just spoke about, it is low code, plus you've got open source or open code underneath that. You can now commit it to Git and you've got DataOps best practices that you can enable in your organization, and and you've got the ability to standardize and reuse components today with Framework Builder and much more coming up down the line. We're going to be talking more about AI in just a bit, so we'll leave that alone. With that, I'm going to go ahead and stop sharing and turn it over to Nathan for a demo.
Nathan
Sounds good to me. Hello, everyone, from whichever part of the world that you're at. Let's go ahead and jump right into the demo as well. Actually, just wanted to start off first just by showing you the Databricks landing page, because for us at Prophecy, we are actually one of the members on their partner, Connect. So whether or not you have access to a Databricks workspace, you can actually scroll down a little bit and you'll find us sitting right under the data preparation and transformation section here. With just two clicks of a button, you can essentially log in and create a new Prophecy account so that you can simply trial it. But as Mitesh will show a little bit later, we also have other forms in which you can engage in a 21-day free trial of our tool as well. With that said, let's go ahead and get started. Switching gears over here, what you should see now is the landing page for Prophecy. I'm going to jump into one of the projects that have prepared for us. This is a Unity Catalog demo that I have. Let me go ahead and open up the project.
Nathan
Awesome. Right off the bat, I'm going to go in and jump into one of the pipelines that I have built out for us. The goal of today's demo, simply put, is I'm going to actually materialize and build out exactly what Mitesh and Roberto described a little bit earlier. I'm going to build out a data pipeline in which we're going to be ingesting some data and writing it into a raw layer that we call the bronze layer. Then I'm going to be doing some joins and then bringing that into the silver layer. Then finally, for the last step, I'll be aggregating the data, doing a little bit of cleanup and reformat, and then writing it into the gold standard as well. These are just basically three levels of data. We call it the medallion architecture or the multi hop approach. Let's go ahead and get started. Right off the bat, as you can see here, this is basically how we go about building out a pipeline within Prophecy. You'll see on the top right over here that I'm currently connected to a Databricks compute cluster. Here at Prophecy, you can think of us as the complete data transformation platform.
Nathan
The way I like to put it is that we're like a really cool Tesla with all the features, but we don't come with an engine. You guys provide the engine, and that engine will come in the form of Databricks in this case. Simply attaching to a cluster, very simple. Then the next thing I'm going to do is essentially build out my pipeline using what we call gems. These little dots that you see on the screen, these are essentially what we call gems. Now, a gem behind the scenes is just a compilation of code that does some function. We grouped all of these different gems into different categories that you see at the top of the page here. For example, under source/target, these are basically, for those of you familiar with Spark, are spark. Reads and are spark. Writes. Essentially, how am I reading the data? And also how and where am I writing the data to? After that, we have our bucket of transformation logic. Within the transformation section, this usually encapsulates 70-80% of all the business logic you'll be doing anyways, whether you're doing aggregates, which you can think of as group-by statements. If you're working with a nested JSON file, we have the flattened schema gem as well that allows you to easily break out those columns into individual columns as well.
Nathan
Then the next section here is that we have the ability to do very simple joins as well as row distributions in a very easy way, but we're able to handle very complex joins as well. For anything that you don't see within these first three sections, you can actually easily build it into the Prophecy tool using our custom gems. For those of you familiar with Spark, Spark is basically the de facto place to do data transformation, logic, and engineering work. There are thousands of libraries out there along with the native transformations. Anything that you can do within Spark, you can do within Prophecy as well. You can either simply write it out as a custom script, just writing down a Python or Scala script, or you can create your own custom gem, which you can think of as simply just a reusable business logic written in Python or Scala encapsulated within one of these components. And then what you can do is you can publish these gems. These gems are all backed by Git, and then they can be used downstream by end users that don't really know a lot of coding, but they are data domain experts.
Nathan
They can use these gems using a customizable UI that you can design yourself while you maintain the actual business logic and the code behind the gem. This is a very powerful value proposition. It speaks to the extensibility of our tool and allows data engineers to give end users a self-service ability to build their own pipelines while working within a standardized guardrail of a good quality code as well. Let's jump right into the pipeline itself. You can simply see here that right now I'm sourcing from two different source gems and writing it to two different target locations. If I expand one of the source gems, for example, you'll see over here, we actually read and write to a very large ecosystems of different sources and targets. This list that you see here is by no means exhaustive. It's simply what we have included here. We categorize it into three sections. Right now I'm reading data, right? I can read data directly from my data lake. This means S3, Azure Data Lake, Google Cloud storage. You can see over here that there's a variety of different file types that I can read from as well. Whether I'm reading from Parquet, CSV files, I'm using Delta.
Nathan
We also support streaming as well. If you're reading and writing into Kafka topics, full support of that as well. Then we have the ability to also read and write directly out of data warehouses as well. Essentially, anything that the JDBC driver can hook up to, we support as well. We've built out a couple of components just due to customer demand with a lot more coming along the way as well. Then finally, and most importantly, we also have the ability to read and write directly into Unity Catalog tables as well. Basically, anything with a Hive meta store underneath it, simply put, if I select Unity Catalog, all I have to do is simply define the catalog, the schema and the table that I want to read and write into, and any access controls that you've defined within Databricks Unity Catalog automatically flows through into Prophecy as well. Then finally, this last section over here is I can bring in the definitions on how I want to actually write out or read this data as well. We make it so that rather than you having to write code, you can simply just pick from the drop down of available properties as well.
Nathan
Now, for the target location here, this is simply just going to be a catalog table. I've already designed it so that it is writing to a bronze database within my dev catalog. This is actually a variable that I can call on to my config just so I can run my pipelines more dynamically. Then this is going to be writing into a customer's table as well. Let's go ahead and run it. Then this is basically going to be the first pipeline. You'll see these blue dots appear within here. These are basically just preview data that you can easily open. It just makes it so that the development experience is so much more easier. Wonderful. Let's move on to the next pipeline. With this next pipeline, I'm actually going to build out a very simple join and just show you how easy it is to just simply drag and drop these different things. The first thing I'm going to do is I'm going to have to pull in the source data. The source data is essentially going to be the same as where I wrote to in my previous pipeline, the bronze tables. But rather than having to recreate things from scratch, you'll see over here that we actually collect all the existing data sets for everything that I have access to within this project.
Nathan
This first pipeline already wrote to this bronze orders and bronze customers' data set. Rather than me recreating it, I could just hit this plus icon and you can see that I can pull this as a gem, either as a source or a target as well. This just makes a life a lot easier. I don't have to go about fishing and finding out where the location of this CSV file is or having to re-input the DBFS or the S3 location in order to get this working. Doing. The next step I do is I can simply just bring in a Join GEM, and as you can see here, I'm just dragging it and it automatically connects. Then I can open this Join gem and start defining how I want to do my join. In this case, my join conditions, I'm going to join it by customer ID. I can just type in here, Customer ID equals customer ID. I can define the Join type. We support all different types of joins. Then if I go into the expressions tab, this is basically the output columns that I want to bring in. In this case, what I'm going to do is I'm going to bring customer ID.
Nathan
Let's go ahead and bring in the first and last name. Notice how all I'm doing is just clicking through a list of things that are available to me on the left. This is basically the available schema that I have at every step of the process. I simply just have to bring in the columns that I want. I'm going to bring in these variety of columns. I'm good to go. One thing I want to note as well is that within every step of this pipeline, every gem, if you will, I can create and write out unit tests just to have little tests running in the background to make sure that I'm testing every gem for its functionality and it's working the way that I want it to work as well. Cool. That's good. Then let me go ahead and bring in the target location, which is going to be a Silver Unity catalog Delta table. I already have it prepared here, so I'm just going to have the plus icon, bring it in as a target, and connect it. You see how easy it was for me just to basically write from two sources, do a simple join, and then write to a target location.
Nathan
But what's going on at the same time is right now I'm looking at the visual component, but if I switch over to where it says code, this is actually where Prophecy offers a lot of value for a lot of enterprises these days. A lot of enterprises, as Mitesh has mentioned, they have a lot of folks that need to create their own data pipelines but don't necessarily know how to code or code very well. At Prophecy, we function as a code-generating platform. Simply put, all of those different gems that you saw me put together, the source gem, the join, and the target gem, what we are doing is we're essentially generating you 100 % open source PySpark Scala code that sits directly inside your Git repository. Each of those gems that you saw earlier and they're just simply Python functions that we've created on the left over here. For example, the bronze gem, which I'm reading from, is simply just a spark. Read. The join gem is simply a. Join and a. Select that I have over here. Then I'm writing it to a silver table. This is going to be a spark. Write. This is typically going to be the work that requires data engineers with years of experience to do, but I did it just very easily in terms of packaging together a couple of gems and wrote out this code for me as well.
Nathan
And all of this code, by the way, sits directly within your Git repository. At no point of this process are we taking this data and putting it into some black box in which you have to depend on us forever in order to use. We are simply just generating the code for you and then giving it to you so that you can keep it within your own Git repository. You can package it and run it through your CICD processes, or you can edit it on your own as well. Cool. I have my Silver Pipeline. I can actually run the entire pipeline, or I can just go up to the specific gem itself and then hit this Play icon. It just makes it so that the development process is a lot easier. You can see your data working along the way as well. This looks good. What I'm going to do next is I'm going to move on to my next pipeline, which is the gold-level aggregates as well. Once this is done running, it should be done running in a second, let's go ahead and jump into the gold-level pipeline. In this pipeline, what I'm going to do is, okay, I already have my silver table, let's aggregate it clean it up a bit, and then write it to a gold-level table.
Nathan
This gold-level table is going to be where all my analysts are pulling out of and feeding into their Tableau, Power BI dashboards, what have you. But I need to prep the data within this tool right now. Let's go ahead and do that. What I'm going to do is I'm going to bring in the silver cable that I just wrote into. This time, instead of a target, I'm bringing it in as a source gem. The first thing I'm going to do is I'm going to aggregate this. What I'm going to do is I'm going to bring in the transform, the aggregate gem. Simply drag it. Once I open it, what I'm going to do is I'm going to group it by customer ID because the customer can have multiple orders. I want to be able to aggregate some of that information and view it by customer IDs. Let's go ahead and go to the second tab, which is group by. One click of a button. I'm bringing in customer ID. Then back to the aggregate tab, what I can do at this point is I can just pick and choose which columns I want. I can just quickly hit the Add All button to bring it all in.
Nathan
But what I'm going to change is that I'm going to give some aggregations to two of the columns here. I don't need order date. I'm going to remove that. Then for Order ID, I want to count the number of orders, so let's go ahead and do Count. Then for the amount, let's go ahead and sum it. Let's go ahead and change the target column names as well. This one is going to be called Total Orders, and this one is going to be called Total Amounts. Hit the Save button. I can just preview this gem over here, very similar to running a cell within the Databricks notebook. I can see the preview data. It looks pretty good over here, but there's a couple of things that I want to change. Okay, firstly, I don't need the other customer ID. This is actually a redundant column. And then also, let's just say I want to also bring in the full name. I don't want to see first and last name. Let's also understand which customers are the most loyal ones. I have the account open date, but what I want to see is the number of days you've been a customer.
Nathan
And then this total amount column, I don't like how the decimals are a little bit wonky. Let's go ahead and round that as well. In order to do that, what we're actually going to do is actually bring in a reformat gem. Let's go ahead and run this one time. A reformat gem, which allows me to just clean up the schema and the data columns a little bit as well. Bringing the reformat gem, I simply connect it. Once I open the reformat gem, it'll show me all the available columns that I have. Let's go ahead and add all of them. But this time, under first and last name, let's go ahead and hit this plus icon so I can insert a column in between. For this one, we're going to call it full name. Now, for those of you that are somewhat savvy with SQL or whatnot, you're probably thinking in your mind, Go ahead and write a concact statement, right, Nate. However, what we can do is we can actually use our Data Copilot, which Mitesh will talk about a little bit earlier, to simply write the code for us. I can hit this Ask AI button.
Nathan
You'll see that automatically infers what I'm trying to do. I already put in full name as the target column, so it's filled in this box to say, give me an expression to calculate the full name. All right, sure. I hit the Enter button and what it does is that our data copilot, which is powered by LLMs behind the scenes, is going to large language models, is going to write out this concact statement. Wonderful. I don't need the first and last name anymore. I can go ahead and remove that. Let's just say now I want to include a column that gives me the number of days that this account has been open for. How long has this customer been around for? What I can do is actually I can write in more of a description as well. This is all prompt engineering at this point. Let's just say, Okay, give me the date difference between when the account opened and today. Hit Enter. Data Copilot right now is taking this prompt, it's vectorizing it, trying to understand what it's doing, and then it's going to write out a SQL expression for me. It wrote it out for me.
Nathan
Let's go ahead and give it an open, close parentheses because that's SQL syntax. Then I'm going to give it a column name as well. This one's going to be called account length days. Wonderful. Total orders is fine, but the next thing we have to do is round up the total amounts column, right? And for this one, I'm actually going to just also open the editor as well to show you that within Prophecy, we have a built out SQL editor, full-fledged with all the available columns here. You can type it in and in each one of these columns does give you the full Wikipedia definition of what it does, as well as examples as well. In this case, what I'm going to do is I'm going to click this Copilot gem, this little icon I have over here, and just type in an expression of what I want it to. I'm going to say round total amounts to two decimal places and enter and see what code it gives me. I like that code, round total amounts to two decimal spaces, hit, Exit. Wonderful. I have my reformats. I've basically done a couple of cleanups.
MiteshI've.
Nathan
Concatenated and give myself a full name. I've created a new column that's going to give me the number of days that the account has been open for. Then I've also rounded up my total amount column to two decimal spaces. Let's go ahead and hit save, and I can also, once again, just simply run this reformat gems just to see how my data looks. Awesome. Ids, full names, the number of days the customer has been around for, and then the total amounts. Cool. Let's go ahead and also do a order by so I can understand which of these customers has been my best customer who has actually contributed to the most from a amounts perspective. I can just bring in order by, GEM, drag it over here, open it, and very simply just choose which column I want to order it by. Let's go total amounts and just do it in descending fashion. Hit Save, run the pipeline, and then generate the sample data frame. You'll see that right now, okay, so Viva is my number one customer with the largest amount of total amounts. This looks great. At this point, I'm going to go ahead and write it to my goal table.
Nathan
I already have that prepared as well. Bring this as a target gem, connect it, and I have a functioning pipeline already. Now, let's talk a little bit about Reusable Business logic. These three steps that I had over here: aggregate, reformat, and order by, maybe these are steps that are going to be done over and over by folks within my platform. Rather than having to reinvent the wheel, why don't we actually make it so that users can easily bring this reasonable business logic with a single click of a button? That's where subgraphs come into play. You can think of a subgraph as a pipeline within a pipeline that can be shared and reused by everyone within this project. What I can do is I can simply highlight these three gems. I can hit this Make Subgraph button, and you'll see that right now it takes all of those three different gems, consolidates it into the single subgraph gem, and when I open it, you'll see that it's all within here. What I can do next is I can go ahead and publish this gem as well. I'm going to call this gem Cleanup, give it a brief description.
Nathan
This is basically it aggregates, it reformats, and it orders. Hit Save, it's published. You'll see over here on the left that this sub graph is actually now available as a reusable component within my project. If I ever wanted to bring it in somewhere else, I can hit this plus icon, pops in just with a single click of a button just like that. Wonderful. Reusable code just available with a single click of a button that I can visually see as well. Again, everything that you see here when I toggle into the code side of things is all available within here as well. This is the final pipeline that I'm working on. This is the read and write gems. Then within the sub graph over here, this is where I've consolidated the aggregate, the reformat, and the order by Python gems as well. At this point, I can actually go ahead and take the work that I've been doing and commit it against my Git repo. Another great thing about Prophecy is that we're encouraging the use of modern best practices from a software engineering perspective. You can actually just very easily, with a couple of clicks of a button, just commit the changes that I've done directly into my local branch and then also merge it to the main branch as well.
Nathan
You see over here that right now, Prophecy shows me everything that I modified. I'm working off of my dev_nathan branch. I can just hit the commit button, and then at this point, all of those changes that I made is committed against dev_nathan. If I wanted to, I could continue with the pull request and then also merge it into my main branch. I'm not going to do that today, but this just goes to show how deeply integrated we are to CICD processes. From a Git perspective, we have support for basically all major Git providers: GitHub, GitHub enterprise, GitLab, BitBucket, Azure DevOps, you name it. What's next? Okay, I like this pipeline. How about scheduling this so that I can rerun this on a recurring basis? When it comes to jobs, it's very easy to schedule jobs within Prophecy as well. We actually support two primary job schedulers. We support Databricks workflows as well as Airflow as well. To create a job, all you simply have to do is hit this plus icon at the bottom, give it a job name. This is going to be called DatabricksJob-Test or called the Dev. Use the Scheduler of Choice.
Nathan
Choose which fabric you want to run against. The fabric is basically a Databricks Workspace in this case, so I have a Dev and a Pro Databricks Workspace. I'll point this one to Dev. I've also defined a couple of default sizes in which I can run my jobs on. I'm just going to pick small in this case, and I'm going to click Create New. You'll see right away that this is a blank canvas for me to now actually give tasks to these jobs. I simply just have to choose from the list at the top or from on the left as well. With three clicks of a button, bronze, silver, gold, I can make sure that they're connected. Right now they are running as dependencies. That means that the bronze job will run first followed by the silver and the gold. I can also actually add additional things as well. If I wanted to add a custom script, this is like a Python script that has to run first in order for the silver job to kick off. I can simply just drag and drop it and make it look like this as well. Delete this for now.
Nathan
Another fun aspect about building jobs within Propthecy as well as I can actually give different cluster sizes to different tasks within this job as well. Let's just say these first two pipelines over here. These are handling a lot meatier data and my requirements are going to have to have a bigger cluster. What I can do is I can highlight those two pipelines, go to Configure a Cluster and for this one, select Large. But then when I finally get to this final pipeline, it's perhaps not as demanding from a resource perspective, from a compute perspective. I can just hit Configure a Cluster and then this one will be small. And again, all of these jobs are basically just running a Databricks jobs API, so it's going to be using the jobs level DBUs. However, I'm just dictating the size of the cluster that I want for each task of my job. At this point, I can either run it interactively like this. I can also flip the switch to have it automatically enabled and scheduled. With running a job interactively, you can actually at this point just go back into Databricks, go into the Workflows tab, go to Jobruns, go to MyJobs, and you'll see that this job is kicking off right now.
Nathan
This is the job I just launched. If I were to go into it and then go into tasks, you'll see that I simply just created a three-task Databricks workflow job. Just very simply dragging and dropping a couple of different options. In about 20 to 30 minutes or so, I basically created a data pipeline that takes raw data, writes it to a bronze location, does a little bit of cleanup and also joins the data and then writes it to a silver table. Then also finally, for the last step, does an aggregate, reformats the data, and then also writes it finally into a gold-level table. I can actually go back to the top-level page and show you that all of this information has been actually captured by our lineage tool as well. You can even see the raw data sets that we have sourced, the pipelines that are wrote into, the following data sets that it eventually wrote into as well, and then all the way down to the gold-level data set as well. Our lineage on prophecy also makes it so that we can capture column-level lineage as well. If I were to let this say, open up the gold data set and say, Okay, I have these available columns under Schema.
Nathan
Let's go ahead and see how the total amount's column came to be. I can actually open up my lineage viewer. Let's go ahead and open up the tabs on the left. I can go into my goal table, expand it, look up the column that I want to find a little bit more lineage about, hit total amounts, and you'll see right off the bat where the total amounts column is located. It's highlighted in bold green that is in the gold dataset, but it was modified under the gold aggregates pipeline, so that's marked in yellow. You can see where it was originally sourced from, which is basically the Silver Level table as well. And all of this lineage information, we make it so that it's available through our metadata API, so you can populate that lineage in any of your preferred tools as well, whether you have Kalibirlation, doesn't really matter. We want to make it so that our tools are simply as open and easy to use and speaks to whatever tools that you have in your ecosystem as well. That was a data pipeline in a nutshell, basically building it out using the medallion architecture, making it so that I'm writing everything as Delta table sitting within the Unity Catalog, govern the atmosphere as well.
Nathan
And then finally scheduling it into a simple Databricks workflow job that then I can promote to be in a production level job as well simply by pointing it to a different Databricks workspace. That's it. I'll pass the microphone back to Mitesh for the next section of today's webinar.
Mitesh
Outstanding. Okay, thanks, Nathan. Let me go ahead and share. Hopefully you can see that. Outstanding demo, Nathan, really appreciate it. There were a lot of great questions rolling in. So, Eonniah, thank you for answering many of those. Some questions about lineage, which you showed at the end, which is fantastic, and some other questions that we'll get to at the end as well. The part about Copilot was quite a hit. That's something I'd like to turn our attention to now, which is the use of AI, large language models within our respective platforms. You see this great quote here from Andre Carpathy, a Tesla and an AI contributor, that the hottest new programming language is English. It turns out both Prophecy and Databricks are using the power of AI and English and natural language to power and simplify many of the aspects of our respective platforms. You saw just a hint of that in the demo that Nathan just showed. We announced this capability just before the Databricks conference in mid to late June. It is really about creating and generating trusted data pipelines through the use of natural language. I think Nathan just showed being able to create expressions using natural language.
Mitesh
There's much more you can do with basically just starting with a statement like compute the average monthly spend for each customer and it will create... It'll give you a starting point for a visual data pipeline that you can then either accept, reject or modify and tweak as needed. It'll suggest transformations and lots more going forward. That's really the power of data copilot within Prophecy to really simplify and democratize in some ways the building or the buildout of data pipelines. There were some questions here about, Oh, this is a Microsoft thing, right? The term copilot, maybe Microsoft, I don't know if they invented it or not, but the way to think about this is Prophecy data copilot is to data engineering as Microsoft's, or in fact, GitHub's data copilot is to software development. We're really using this for generating visual data pipelines. Microsoft is using it for software development and code, but same idea, leveraging AI within our platforms. Databricks also has an AI system, and would love to turn it over to Roberto to talk a bit more about that and the power of both Prophecy Data Copilot and Databricks AI Assistant.
Roberto
Thanks, Mitesh. Yeah, Databricks Assistant, we had some exciting announcements about this during our data and AI summit. Basically, as Mitesh was indicating, a context-aware tool that's basically useful for helping you generate code snippets that is also context aware, meaning that it's aware of the logic in your cells, in terms of the metadata that you have residing within Unity Catalog, and it uses all these inputs to provide the most tailored output possible based on the whole context in which you're trying to write your pipeline. So, yes, it generates actual logic, leverages you need to catalog as the cash metadata to produce the most tailored response possible, and it's available anywhere in the platform where you would be presumably writing code. So within the Databricks notebook, for example, if you're writing some Python, some Scala, some R within the SQL editor, if you're writing some ad hoc SQL queries. So all this is part of our Lakehouse IQ suite of tools to really make it easier for these organizations to rise on the maturity curve that we talked about earlier and become more AI predictive modeling companies rather than companies that just focus on historical analytics. Next slide, please.
Roberto
Okay, great. Yep. Just as the tech was saying, English is the new preferred programming framework. So being able to just write natural language and write English, similar to how you interface with OpenAI or ChatGPT or other LLMs out there, the same principles of using natural language, but again, very much tailored to your context and your environment. Similar algorithms, but the inputs are different because inherently you're doing different things within the context of the pipelines that you're building and you're optimizing for your business with different metadata. So the assistant will account for all those different variables and factors. Then really useful for a wide range of things. I wouldn't say plan to just cut your development staff and just use the assistant to write all your code. It's more so meant to generate templates that you use to expedite the amount of time taken to write a new pipeline, or if there is a particular function where you're not familiar with the syntax, it's more meant to augment and reduce manual, janitor coding tasks than it is to write your next full end-to-end production pipeline. I would say we're not at that level of advancement yet, maybe 10, 15 years down the line.
Roberto
So that's essentially what the experience of the assistant is. It's in public preview for all Databricks customers. So that little widget there on the side shows what you can do, but feel free-to-play around with it and get a sense for not only the outputs it delivers, but just the best way to prompt it, because there are certain ways that are more likely to get the outputs that you're looking for. There's a lot of inputs and metadata we can use, but ultimately there are certain best practices for how to prompt to get the optimal output. Next slide, please. Okay, great. As Mitesh was saying, very much a better together narrative as it pertains to our assistant and the data proxy copilot. Databricks being historically a code first platform, our assistant is optimized for generating SQL and Python code and then writing that code within a notebook, within a SQL ID, within a file, within the abstractions that if you're a code first data engineer, data analyst, data scientist, like the familiar workflow that you follow today, allows for auto complete, fix issues, syntax, generating snippets, looking at different queries that are based on tables that exist within Unity Catalog, whereas Prophecy Copilot more so meant for that visual DAG interface, so building those pipelines visually, being able to do the drag and drop, the GUI-based things for the low coding data engineers.
Roberto
So very much complementary. Some intersect, but not a ton, more so very much complementary. So obviously, Copilot meant to generate those pipelines, suggest your transformation generates beautiful visuals, documentation, lineage, all those kinds of things. So yeah, Mitesh, I'll pass it back to you in case you want to add anything on that front.
Mitesh
Perfect. You covered it really well. Thanks so much, Roberto. Better together. Love it. The power of two very innovative companies leveraging the power of AI to simplify tasks in our respective platform. So amazing job. Okay, this is coming to the conclusion of our webinar here, I wanted to basically wrap things up with a tale from the field section. Again, we've got Roberto and Nathan who are in the field, what we call the field, talking to prospects and customers every single day about pain points, issues, challenges, and would love to kick things off with a bit of a Q&A. Before I do that, for the, I guess, more business folks in the audience, we want to point out there was a lot of technical content in this webinar thus far. All of this, again, is meant to democratize the power of building pipelines, transformations with prophecy, but ultimately for business impact. What is that impact? We've had a number of companies in a range of industries leveraging for some incredible business outcomes. Taking sports and baseball as an example, analytics and data is very important in the world of sports. Maybe it started with Moneyball, but in this particular case, this is in fact, in the case of Texas Rangers in baseball, where they had very rigid siloed architectures, limited data engineering resources, all the pain that we spoke about that Prophecy and Databricks are addressing together, they had that pain before moving to privacy and Databricks.
Mitesh
With the combination of our two platforms, we've had some incredible measurable outcomes here with going from one week down to a day for pipeline development, three times more analysts and developers building pipelines, and 10 times faster, meaning stakeholder KPI. Some great outcomes here from the Texas Rangers. We've got a great webinar that's available for replay if you want to dig into that more. Then Waterfall asset management, completely different industry, financial services here, managing complex financial assets. Similar pain points early on. Then after moving to Prophecy and Databricks, you can see some of the incredible measured outcomes here at the right with going from, I think, three weeks down to a half a day in terms of team productivity and their workflows, and then 48 hours down to two hours with their time to insight. Again, great business outcomes here across a range of industries. With that, we're going to start now moving into Q&A. I'll kick things off here just to crease the skin, so to speak, and then we'll get into some of the Q&A here from the audience. So let me kick things off. We're going to bucket these into just a few different categories, starting with the partnership.
Mitesh
Roberto, you spoke a bit about the complementary nature of Prophecy Data Co-Pilot and Databricks AI System. What about the company level and or the broader product level together? What are your thoughts on the Prophecy and Databricks partnership?
Roberto
Yeah, it's a great question. Thank you, Mitesh. Yeah, so as Nathan highlighted, Prophecy is natively available within our partner, Connect Ecosystem, which is a lot of the most important and most strategic partners that Databricks has. So meant to make it as simple and as easy as possible for Databricks customers to sign up for Prophecy to kick off a free trial and to start leveraging the platform. That's very much like a strategic partnership thing that we're doing in terms of a better together motion. And historically, Databricks being a platform that's more suited to a code-first persona, code-first data engineers, data scientists, data analysts. Prophecy is a perfect complementary partner that caters to a different audience that's, as Mitesh was saying, democratizes the ability to create these pipelines, to iterate quickly and to deliver value to the business. We see Prophecy obviously as a very strategic partner in terms of reaching those audience that historically probably wouldn't use Databricks in the past. Yeah, so to address the question very much better together, like strategic partnership that we see on our end.
Mitesh
Okay, thanks, Roberto. Nathan, same question for you. It turns out, Nathan, you actually came from Databricks before Prophecy. Is that right? Do you want to talk about this as well?
Nathan
Yeah, that's right. The way that I see it, and I was a customer success engineer during my days at Databricks, and one of the toughest hurdles to sometimes overcome is just simply just having to code. Oftentimes what we notice is that when we want to evangelize and bring the power and leverage the power of Databricks to a much wider audience, there is a little bit of a learning curve for folks that may be data domain experts but are not really familiar with how to code in Pi, Spark, Scala, let alone SQL. The vision that we have at Prophecy is to make it so that everyone can take advantage of their own data without having to be coding Ninjas as well. And that's actually what I really saw when Prophecy was introduced into some of my accounts as well as some of my colleagues accounts as well. The adoption rate was just so much faster because now you've enabled a large ecosystem of users who did not come from a data engineering, let alone computer science background, but are now able to create the same level of complex and data revolutionizing pipelines essentially that a 10 year data engineer veteran would do.
Nathan
And when it comes to the code generated, I've had my years at Databricks. I can put a stamp of approval that the quality of the code is pretty much equivalent to what we would be writing manually as well.
Mitesh
Outstanding. Okay, great perspective from you, Nathan, having now come from Databricks and now Prophecy. Thank you for that perspective. I think we have just a few minutes left, so I'm actually going to move on to just a couple of common questions maybe for you, Nathan, and then we'll see if we have time for audience Q&A. If we don't hear, we'll certainly follow up separately. Before we kick this off, I asked you, what are some common questions, Nathan, that we're hearing from customers and prospects? I'll start with one of them. There's a lot of talk about low code here, and people might assume that low code means limited in some way. Your thoughts on that concept that low code is in fact limited?
Nathan
Yeah. I saw this actually comment pop up a couple of times into our group chat, and let me assure you we are not taking away your jobs, data engineers. In fact, we are enhancing your life because Prophecy is essentially a productivity tool that allows both data engineers as well as data analysts to do their jobs better. For data engineers, rather than having to answer to a litany of queries from downstream analysts to build this pipeline, build this pipeline, build this pipeline, what you're essentially doing is you're now actually creating frameworks and reusable and standardized templates to ensure that downstream users are more than capable of building their own pipelines, but within a predefined guardrail of standardized code that you, as a data engineer and your team, has built. This code is all backed by Git. It's owned by you, and you can also publish it and versionize it as well. This way that you can have those little custom gems that have multiple versions. If you release a new version, it doesn't screw up anything in production, but the point is that from a data engineering perspective, your role now becomes very strategic in a sense as well.
Nathan
How can I best democratize my data to the entirety of my organization rather than having to bog my team down with all of these one-off requests from various downstream teams as well?
Mitesh
Fantastic. Okay, that was a two-for-one. You answered both, hey, low code is not in fact limited in the case of Prophecy, as well as the data engineers and the more technical folks have a more expanded role and are able to focus on the higher value tasks. So perfect. I do want to get to this. There were some questions as you were going through the demo, Nathan. How can I get my hands on this? Is there a free trial? Of course, you can spin it up through Databricks Partner Connect. If you would like, click... Well, you can't click on this link, but we're going to drop it in the chat. Someone will drop it in the chat and/or you can simply take a screenshot of this or take a picture of this on your phone and it'll lead you to a 21-day free trial. I can attest to the fact that you will learn a lot. And most importantly, it's actually a pretty fun, free trial to go through, so I highly recommend it. I think we just have a few seconds left, and I'm probably going to have to refresh my deck to go through questions.
Mitesh
In the few seconds we have left, let's maybe just go through one... Oh, boy, we're at time here. I'll go through the first one and then we'll follow up on the rest separately. Different from Alteryx and Matillion. I'll just bucket this and say legacy, WillCode solutions. I spoke about this earlier, but the biggest difference here is two or three different things. One, in some cases, and I'm not going to name names here, in some cases, you actually have to pull the data into your desktop and/or a laptop and do your transformations there. It's very limited to small data in some ways. It's not cloud native. It's not native to the cloud environment. The second big piece here is that in many of these low-code legacy tools, the visual environment underneath that is proprietary code. It is not open code that you can just take and modify, commit to get, leverage for DataOps, create tests out of, et cetera. It is proprietary code underneath and therefore locks you in. The third piece being, I didn't mention this, but they are rigid in that they are not extensible in the ways that Nathan described earlier. With that, I'm going to go ahead and stop the recording.
Mitesh
We are at time. We'll get to the other questions separately. Apologies, we ran out of time here. But Nathan and Roberto, thanks so much for participation here. Thank you all for joining the webinar. I appreciate it.
Speakers
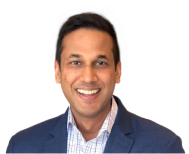
VP of Product Marketing
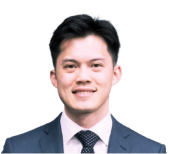
Sales Engineer

System Architect

Ready to start a free trial?
Modern enterprises build data pipelines with Prophecy
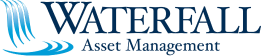
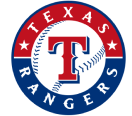